The University of Rhode Island (URI) has been using Eli Review continuously since Spring 2012. Previously, we’ve written about how Program Director Nedra Reynolds has led faculty from across the disciplines to make formative feedback a priority in their courses.
Coinciding with this curricular change effort is an innovative research and evaluation program led by URI faculty member Ryan Omizo. Ryan is taking advantage of the access Eli Review provides to both student texts and student performance data to study student learning.
We recently interviewed Ryan to learn more about his contribution to the presentation at #4C16: “Eli Review as Strategic Action,” and our own Bill Hart-Davidson offers some commentary on how Ryan’s computational approach is important to writing studies more broadly.
Ryan, the team is helping students become scientists by teaching feedback and revision. What genre is the group working on now?
Ryan Omizo: Right now, our pilot study focuses on writing pharmacy SOAP notes. Students are learning to recognize what each part of the SOAP note should include as they review peers’ drafts and revise their own.
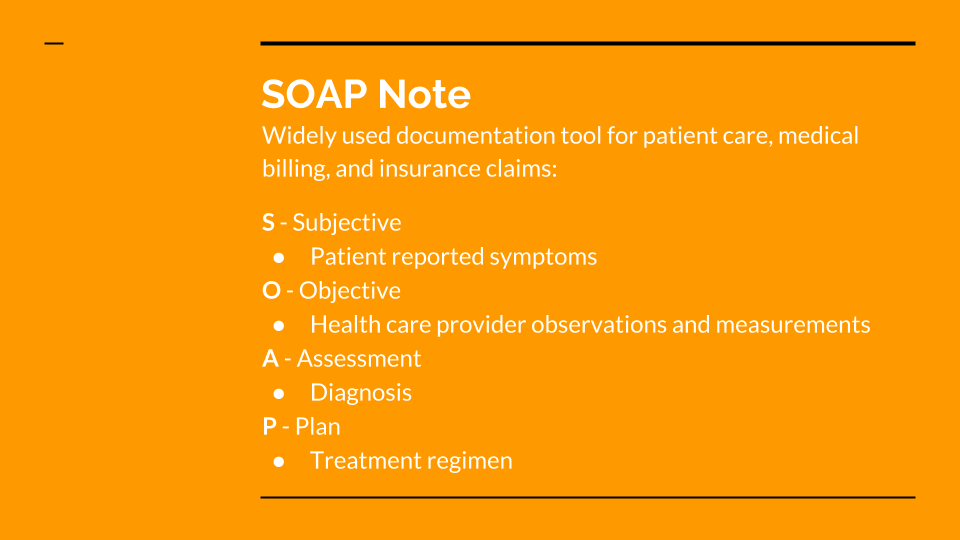
Bill Hart-Davidson: A SOAP (Subjective, Objective Assessment Plan) note is a common form of writing for health care providers. You can think of it as the writing care providers do for a patient’s medical record. The main goal of a SOAP note is to document what forms of care a patient has received and should receive. Care providers compose SOAP notes both for historical purposes and also to coordinate care decisions with other providers.
You had used Eli prior to going to URI, so you had a sense of what data in the system could be used to conduct research. What research goals did the team set as this particular project began?
RO: We wanted to study reviewers’ comments in more depth. The chief goal of our pilot study is to establish a baseline that might show a link between the quality of feedback given and how this feedback explicitly deploys the language of the prompt as guiding action for the writers under review.
BHD: Ryan and the URI team is working to test an assumption that many writing instructors have, which is that over time students begin to develop language to talk about their writing. This “metadiscourse” helps writers to be more aware of the goals they have and the means they can use to revise a piece of writing. This language appears in the assignment prompts and criteria that teachers provide students. So, the theory is that if students’ knowledge of how to self-assess is improving, we’ll see more similarity over time between the way they talk to one another (in review comments) and the way their instructors talk to them about writing (in review prompts).
So, this project examines the extent to which reviewers uptake the discipline-specific language in the review prompt when giving feedback to each other. How’s that research possible?
RO: Eli Review’s comment digest download allows us to collect raw comment data and see the relational links between reviewers and writers for each review session. The comment digest lists who told what to whom, so we can use that information to visualize different aspects of the peer learning network. For example, using different analytics, we can see how many comments were exchanged, how long the comments were, and even what those comments were about.
What kinds of analysis have you done so far?
RO: I have conducted exploratory analysis using comment word count and cosine similarity scoring between the language of the review prompts and the language of feedback given. (missing sentence about the value of word count analysis)
I realize this flies in the face of many of the maxims we espouse in the writing classroom about quality over quantity or content over paper length; however, at a fundamental level, the difference between an extensive review and a superficial review comes down to the length of the review. In the very least, a longer reviewer has more potential to provide probative feedback because it gives the recipient more to work with. This is the value of the “word count” metric. Although a blunt measure, tracking word count can give instructors a rough baseline to approach reviews.
What you describe as “uptake of discipline specific language” can be measured by cosine similarity.
RO: Cosine similarity can test the linguistic proximity of two documents by comparing the dot products of two term frequency matrices. For the pilot study, we are comparing the linguistic features (words present or absent) of the review prompts to student feedback.
We don’t want you to give away all you best spoilers, but can you tell us one of the things that you’ve found that has been surprising or helpful?
RO: Cosine similarity scoring has been most interesting. This measure compares documents and gives us a number expressing how many words are used in both documents. In information retrieval searches, the meaning of this number is well understood: are two documents related or not? For peer review, the notion of similarity is not cut and dried. We expect large differences between prompts and feedback because students are responding to specific, unique drafts; we expect students to use prompts as launching points.
In light of this, we need to first establish a range of similarity scores and correlate these ranges with a qualitative judgment on the review itself. For example, feedback that is more similar in language to the review prompt is considered a better review by instructors and peers. Or, feedback that falls within the lower ranges is considered less helpful by instructors and peers.
The similarity scores that we have found during our pilot study lie within consistent percentile ranges, suggesting a pattern. For example, there is a wide discrepancy between the similarity scores in the top percentile (1.0 – .75) and the bottom percentile (.25 – 0.0). When looking at the bottom quartile, we can qualitatively note the differences between the two regions, meaning that we can establish a baseline metric to judge reviews.
BHD: Ryan’s work here, as he notes, is foundational because, if we want to measure change over time, we have to grasp where students begin. The results Ryan has reported in his team’s initial work suggest that a baseline is measurable using the cosine similarity metric.
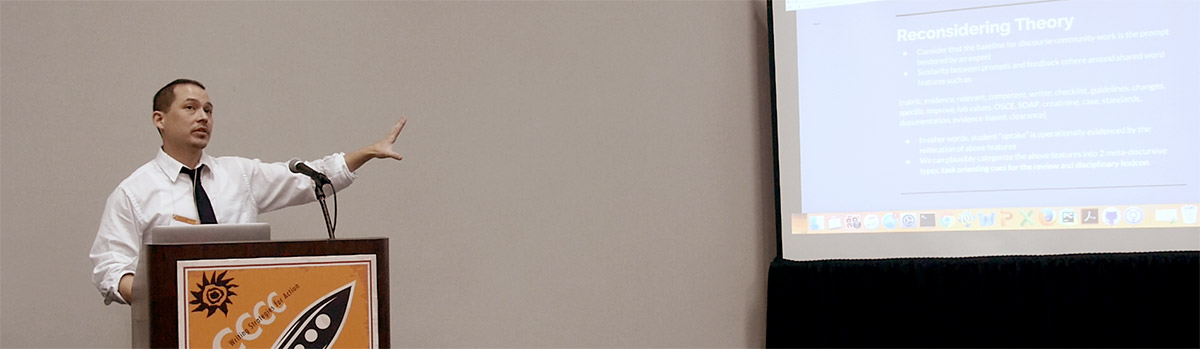
What new ground do you think this work explores for you and your colleagues at URI and for the broader field of writing studies?
RO: This study furthers the goals of Eli Review to conduct evidence-based research into peer review and student learning. I think our use of computational analytics is another step towards grounding the understanding of peer review in empirical data, not just intuition. We also feel that this work is on the way toward offering users of Eli Review (instructors and students) rapid, automated feedback for goal and task correction.
For the field of writing studies, I think the most notable insight that I have received doing this work is that we may have to return to naive questions about how writing and review works.
What do you mean? Have you found something more simple than cosine similarity that helps instructors coach more effective peer reviews?
RO: Simply thinking about word count as means to assess the quality of peer review writing is a dramatic departure from the prevailing theories of writing in the field. Issues such as length of writing is often treated as ancillary to the development of ideas or a writer’s individual voice. In other contexts, word count or document length is used as a means to enforce disciplinary rigor. However, word count can be used to conduct assays into the content of a review because word count delimits the possibilities of a text. You can only say so much in a three word review. You can say much more with a 20 word review. And these distinctions can be immediately and automatically assessed and alert instructors that an intervention may be needed.
BHD: On the Eli team, we often talk about helping teachers find “indicators” of student learning. Ryan and his team are doing great work to validate some new indicators for us that may well become instrumental to Eli’s analytic reports in the future. What is sometimes surprising is how seemingly simple things like word count can be powerful indicators when viewed in the right context. For example, the difference in word count between 5 and 20 words vs. 30 and 50 words is 20, but what a difference!
Because we are focused on formative feedback at Eli – giving teachers and students information that they can use to improve their writing ability – both the timing and the other information we present in our feedback displays matters a great deal. So while we would never think about word count by itself as particularly interesting, we can see through analysis work like Ryan’s and like that of our own Melissa Graham Meeks that some *ranges* of word count are useful in helping us to pinpoint where students may be in need of additional help.